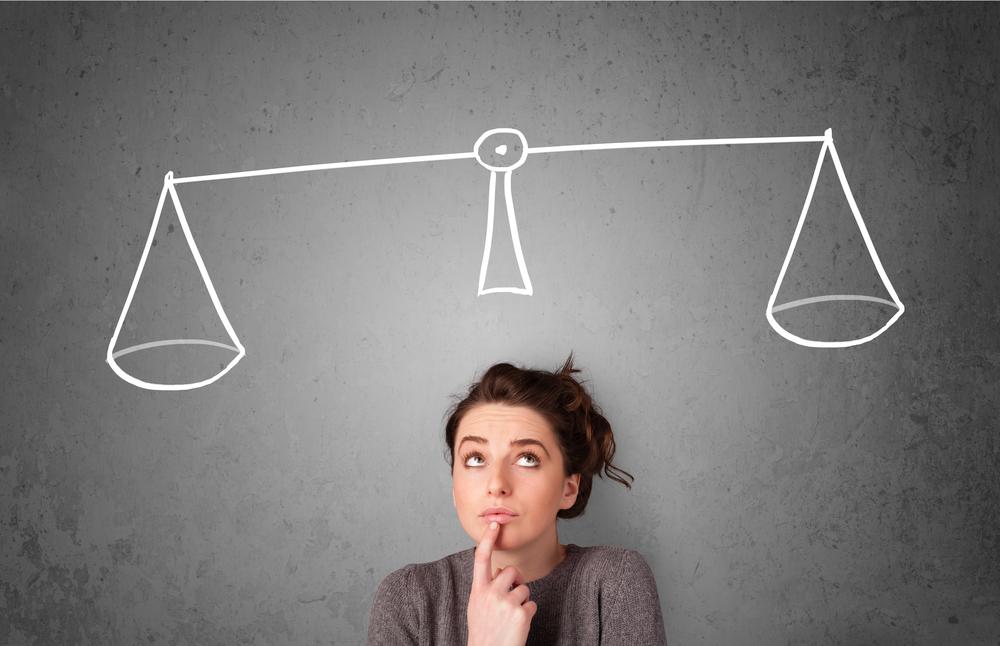
How Much in Stock vs Bonds?: A Tactical Approach
In an earlier article I introduced the concept of using current market conditions to tactically rebalance between stocks and bonds around a long-term strategic asset mix. This methodology I call the “Dynamic Model” approach to managing the asset mix. In this article, I will explain the model’s methodology and compare its' back-tested performance to a static 60/40 stock/bond asset mix.
Use the Expected Return Spread to Over- or Under-Weight Stocks vs Bonds
The basic premise of the Dynamic Model is that expected return spreads between two asset classes can be used to allocate assets between them. Furthermore, it is reasonable to expect a modest increment of return enhancement and/or risk reduction from the use of this methodology over time compared to a static asset mix that exactly matches the strategic allocation.
Such a proposition may seem obvious and straightforward, but in fact it is quite controversial in some circles. Specifically, proponents of market efficiency1 will argue that if stocks are priced to provide an unusually high expected return relative to bonds, there are reasons for this. For example, stock risks may be higher than normal, or bond risks lower than normal.
No doubt changes in risk perceptions have an influence on pricing. In fact, proponents of behavioral finance2 will argue that such changes in risk perceptions are one of the major contributing forces that drive prices to deviate from their fundamental values. People set market prices and people are fraught with all kinds of behavioral biases that have been well documented. In brief, they are short-sighted emotional creatures that allow their feelings to influence their buying and selling.
Most honest observers would admit that volatility in market prices far exceeds any reasonable amount of volatility in the underlying fundamentals that should (theoretically) determine those prices. For example, investors were “irrationally exuberant”3 during the internet bubble era of the late 1990s. Arguably, they were “irrationally despondent” at the bottom of the Great Recession. Wise investors with deep pockets and long time horizons may be able to take advantage of extremes in investor emotion. Warren Buffet is famously quoted saying “we simply attempt to be fearful when others are greedy and to be greedy only when others are fearful."4
The Dynamic Model described here does just that. Importantly, all of the inputs to the Dynamic Model are objective and quantifiable. No subjective guessing. The system described here is a very simple and intuitive, but it has worked well historically, and its simplicity makes it easily implementable.
The basic idea is to compare the current expected return spread of stocks and bonds to their historical average return spread. If stock prices are high and expected returns are therefore low relative to bonds, stocks are under-weighted. If stocks are cheap and their expected returns high, they are over-weighted.
What follows is a monthly back-test of the Dynamic Model covering the time period 12/31/1999 to 12/31/2018. To keep things as simple as possible, I use the S&P 500 to represent stocks and the Bloomberg Barclays US Aggregate Bond Index to represent bonds. The methodology could easily by applied to other indexes, and perhaps I will document those tests in the future.
The data points required are few: 1) monthly stock dividend yield and dividends per share (DPS) starting 12/31/1979, 2) monthly bond yield-to-maturity starting 12/31/1989, and 3) monthly returns for the stock and bond indices starting 12/31/1999. The reason that 20 years of DPS history is required before the first return observation is as follows. The first 10 years of DPS history is used to establish the long-term growth rate of stock dividends per share. Starting 12/31/1989, this growth rate is added to the dividend yield to derive the expected return for stocks. Bond expected return (YTM) is then subtracted from stock expected return to calculate the expected return spread starting 12/31/1989. Starting 12/31/1999, the then-current expected return spread is compared to its 10 year exponentially-weighted moving average to determine the extent to which the current spread is above-average or below-average.
Some researchers prefer to use averages based on as long a time period as the data will allow. I believe that markets are dynamic and ever-changing. The older the observation, the less likely it is to be relevant to today. Using 120 months (10 years) of data provides a large enough sample period to provide some data stability but avoids using data that is so old that it is less likely to be relevant.
I always use exponentially-weighted moving averages when doing time-series analysis. The idea is to give more weight to more recent observations, and less weight to older observations. I think this tends to more accurately reflect how past events influence current expectations, and also it makes the analysis much less sensitive to the oldest observation dropping off each month as the data gets updated. The graph below shows the weights I use for a trailing 120-month (10 year) time period.
The history of the three inputs to the expected return calculations are shown below. The bond yield (blue line) generally declined until about 2012, then flattened out for several years, and recently began to climb. Stock dividend yield (orange line) declined throughout the 1990s from about 4% to about 1% as stock prices increased faster than dividends but has been fairly steady at around 2% since then, except for the spike during the Great Recession. 10-year dividend growth rate (gold line) has been influenced by both economic and market cycles. It declined during the 1990s, bounced back after the Internet Bubble burst, collapsed following the Great Recession, but then quickly bounced back and has been around 5%-7% since.5
The expected return for stocks is estimated by adding the dividend yield to the dividend growth rate. The yield-to-maturity for bonds is subtracted to get the stocks-bonds expected return spread (blue line below). However, we need some way to answer this question: is the spread higher or lower than normal? What is “normal” can be measured in a variety of ways. As mentioned above, I use the 120 month exponentially-weighted moving average (EWMA). This methodology is better able to incorporate the changing nature of what is “normal.” The “normalized” spread subtracts the 120-month EWMA from the current level. The result is the orange line below. It is this normalized spread that I will use to calibrate how much to over- or under-weigh stocks vs bonds.
Calibrate the Dynamic Model
The next task is to translate the signals from the normalized spread into stock and bond allocations. My objective is to compare the Dynamic Model to a static 60/40 stock/bond mix, so it is important to calibrate the back-test so that the average stock allocation for the time period tested will be 60%. The next task is to determine how much to add to or subtract from the average for each 1% of normalized return spread. I chose a multiplier of 10, so that an additional 10% stock allocation would be added for each additional 1% in normalized expected return spread of stocks over bonds. The resulting asset mix history is shown below.
Note that the overvaluation of the stock market at the height of the internet bubble was reflected in the low expected return of stocks relative to bonds at the start of the study period, resulting in a very low stock allocation of only about 20%. After the internet bubble burst, the spread grew steadily. Going into the Great Recession, stock expected return was high relative to bonds because dividend growth had been strong. As stock prices declined during 2008 and early 2009, stocks became even more attractive. Then, as corporations reduced or eliminated their dividends, the dividend growth rate plummeted, driving down the expected return for stocks and reducing the stock allocation to about 45% in early 2010. Then dividend growth strongly rebounded, driving up stock expected return and increasing stock allocation to a peak of about 95% in late 2012.
The graph below shows the cumulative return of the Dynamic Model compared to the static 60/40 approach. As expected, the low stock allocation at the start helped protect against the bursting of the internet bubble. However, the approach suffered during the Great Recession, since the stock allocation was fairly high going into the crisis. Since then, the model has performed well. A more stable and robust methodology for estimating stock dividend growth rates would have substantially enhanced performance, but I have opted to keep things as simple as possible.6
As shown in the return/risk graph below, for the overall time period, the Dynamic Model had a return of 7.0%, compared to 5.4% for the 60/40 benchmark, for a return difference of about 1.6% per year. Risk was roughly comparable between the two.
How realistic is this back-test? The methodology I have described is fairly simple, perhaps even simplistic. (In practice I use several other factors and a more complex methodology in forecasting expected returns. I also include a larger number of asset classes.) Beginning the test on 12/31/1999 is somewhat arbitrary, but it does capture two severe market downturns. More extreme market conditions make for more extreme performance results. Also, using a multiplier of 10 to calculate the target stock and bond allocations (+/- 1% normalized return spread = +/- 10% stock allocation) results in fairly dramatic extremes in asset allocation. Less aggressive implementations using lower multipliers would have resulted in commensurately lower returns relative to the 60/40 benchmark.
There may be good reasons for throttling back on the multiplier. Below is a graph showing the cumulative rolling 12-month return difference between the Dynamic Model and a static 60/40 mix. I noted above that the Dynamic Model performed very poorly during the Great Recession. At its nadir, the 12-month return difference was -8.4%. Not everyone can live with that level of potential under-performance relative to a benchmark. Cutting the multiplier in half from 10 to 5 would cut the return the return spread in half (from 1.4% to .7%), but it would also keep the allocations 50% closer to the 60/40 benchmark and cut the return difference compared to the benchmark by half.
Regardless of how the model is calibrated, this simple methodology for dynamically implementing a tactical target asset mix could potentially have at least two important benefits for investors. First, by taking advantage of the expected return information provided by two simple and readily available fundamentals (bond yield-to-maturity and stock dividends per share), I have shown that it is possible to potentially add a noticeable increment of return. Perhaps even more important, however, is the discipline that such a system provides. Having a theoretically defensible and empirically proven methodology to follow can take investor emotion out of the decision-making process, which may be the most important benefit of all.
1The Efficient Market Hypothesis asserts that prices reflect all available information. Thus, any attempt to profit from a perceived mispricing will be useless. Over the past 30 years, the weight of academic evidence in favor of at least some level of market inefficiency has persuaded most observers that markets are not perfectly efficient.
2Behavioral finance is something of a cross between finance and psychology. Classical economics assumes that everyone always behaves in a rational manner. Behavioral finance relaxes this assumption and studies how people actually behave under conditions of uncertainty. This discipline has documented a number of biases that are quite common. For example, “herd behavior” can contribute to financial bubbles or panics. Barberis and Thaler (the latter won the Nobel Prize in Economics in 2017 for his work in behavioral finance) have done an excellent summary of the literature on the subject.
3”Irrational exuberance” is a term made famous when used by Alan Greenspan in a 1996 speech cautioning investors about the growing valuations being applied to dotcom companies with no earnings and little prospect of any. In 2000, economist Robert Shiller used the term as the title for his book arguing that the stock market had become overvalued.
4Warren Buffet quoted in a Berkshire Hathaway annual report.
5What causes the ebb and flow of dividend growth? Partly, it is the cyclicality of corporate profits. Earnings are much more volatile than dividends, of course, (which is one reason for using dividends rather than earnings as a fundamental barometer of expected growth), but expectations regarding future profit growth greatly influence the willingness of corporations to increase their dividends. Another influence is variation in inflation rates. (I am measuring nominal growth in dividends.) Corporations typically try to raise their dividends to at least roughly keep up with inflation if possible. Also, growth in dividends per share (DPS) may also be indirectly affected by net stock buybacks—as share count declines DPS grows commensurately if corporations spend the same aggregate dollar amount on dividends.
6The Dynamic Model’s results are sensitive to variations in the method for estimating growth. I have used the 10-year compound growth rate in DPS in live models for years, but for more stable and robust results I blend it with forecasts of expected real GDP growth and expected inflation derived from the Treasury-TIPS spread. For the purposes of this article, I kept it simple and used only the DPS growth rate.