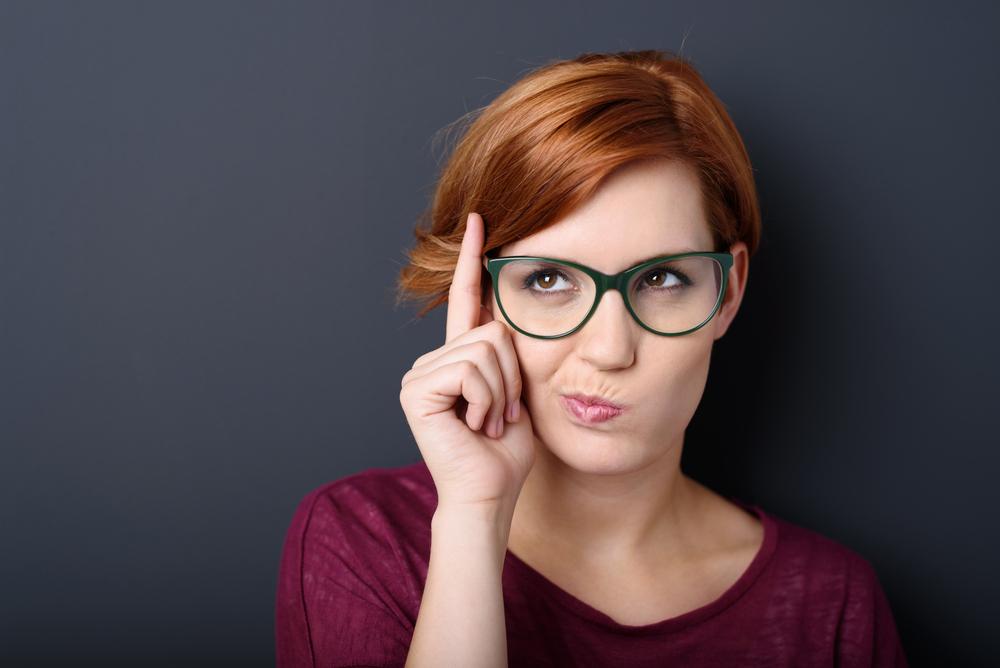
How Much Interest Rate Risk in Bonds?: A Tactical Approach
Use the Expected Return Spread to Over- or Under-Weight
The basic premise of the Dynamic Model is that expected return spreads between two asset classes can be used to allocate assets between them. Furthermore, it is reasonable to expect a modest increment of return enhancement and/or risk reduction from the use of this methodology over time compared to a static asset mix that exactly matches the strategic allocation.
To illustrate the application of the Dynamic Model to managing interest rate risk, the benchmark will be a 50/50 blend of the 3-month Treasury and the 10-Year Treasury. The basic idea is to calibrate the allocations between the two using the normalized yield pickup of the 10-Year Treasury over the 3-month Treasury, defined below, since for bonds, the yield is the expected return.1
The graph below illustrates the concept of using the amount of additional expected return (“yield pickup”) available in a higher risk bond segment to calibrate the amount to invest in that segment. Within the bond market, risk could be defined as interest rate risk (e.g., 10-Year Treasury bond vs. 3-Month Treasury bond), credit risk (e.g., high yield bonds vs. investment grade bonds), or currency and political risk (e.g., emerging market bonds vs. U.S. Treasury bonds). As shown in the illustration, higher risk normally offers higher return. The gray line in the graph is the average yield pickup, which in this illustration is 1% (2% yield for higher risk and 1% yield for lower risk). When the yield pickup is higher than average, as illustrated with the orange line, a higher allocation to the higher risk segment is called for. When it is lower than average, as in the case of the blue line, a lower allocation to the higher risk segment is in order. Note that in absolute terms, the higher risk segment still offers a higher yield, even when it is well below normal. This is generally the case—higher risk segments almost always offer a higher yield. The key is the “normalized”2 yield pickup (current yield pickup minus historical average yield pickup), which in the case of the blue line is -.5% (0.5% current pickup minus 1.0% average pickup).
The historic yields for the two bond indexes are shown below. The 3-Month T-Bill Index yield (blue line) reflects Federal Reserve monetary policy, which traditionally has been limited to the short end of the yield curve. Note the fairly dramatic swings in rates, indicative of the Fed attempting to either slow or jump start the economy. For example, after the crash of the internet bubble in 2000, the Fed aggressively pushed down interest rates for several years before gradually raising rates starting in mid-2004. Short-term rates began to fall again in late 2007 and the Fed drove them to nearly zero by late 2008 and held them there for the next several years. Finally, in December 2015, the Fed began to steadily raise rates in .25% increments.
The 10-Year Treasury Index yield (orange line) followed a roughly similar trajectory, although without the dramatic lows seen in the 3-month index. Not satisfied with the results of its “zero interest rate policy” on short-term rates in the aftermath of the Great Recession, the Fed began to aggressively buy up longer-term Treasury and mortgage-backed bonds under its “quantitative easing” policy, driving down their yields, in an effort to boost the economy, particularly the housing market. In September 2017, the Fed announced that it would begin to “unwind” its enormous holding of Treasury and mortgage-backed bonds by allowing them to mature without replacing them, a process that will take years.
In order to use the yield pickup between the 10-year and the 3-month bonds to make an allocation rule between them, we need some way to answer this question: is the yield pickup higher or lower than normal? The “normalized” yield pickup (orange line at right) subtracts the trailing 120-month exponentially-weighted average from the current level (blue line). It is this normalized yield pickup that we will use to calibrate how much to over- or under-weigh 10-year vs 3-month bonds.
Calibrate the Dynamic Model
The next task is to translate the signals from the normalized yield pickup into allocations. The present back-test will compare the Dynamic Model to a static 50/50 10-year/3-month bond mix. Therefore, I calibrated the back-test (which begins on 12/31/1999) so that the average allocation for the time period tested would be 50/50. The final task is to determine how much to add to or subtract from the average for each 1% of normalized yield pickup. I chose a multiplier of 15, so that for each additional 1% in normalized yield pickup an additional 15% 10-year T-bond allocation would be added. The resulting asset mix history is shown below.
Note that the pattern of allocation was roughly the same for both of the recent Fed easing cycles: more of the 10-year T-bond was owned early in the easing phase to reflect the steeper yield curve that generally resulted. Then, as short-term rates were allowed to rise, more of the allocation shifted over to short-term bonds.
The graph above shows the cumulative return of the dynamic allocation model compared to the static 50/50 approach. For the overall time period, the Dynamic Model had a return of 3.7%, compared to 3.3% for the 50/50 benchmark, for a return difference of about .4% per year. As shown below, the standard deviation was slightly higher for the Dynamic Model, but as shown in the next graph, most of the volatility in the return difference between the two was “upside volatility”—not really risk at all.
The graph below shows the cumulative rolling 12-month return difference between the Dynamic Model and a static 50/50 mix. The worst period was right after the bursting of the internet bubble, when having a higher allocation to the 10-year bond would have resulted in a higher return. After that, however, the Dynamic Model performed exceptionally well, with only a few, brief, shallow periods of under-performance.
This simple methodology for dynamically managing interest rate risk could potentially have at least two important benefits for investors. First, by taking advantage of the expected return information provided by a simple and readily available fundamental indicator (bond yield-to-maturity), I have shown that it is possible to potentially add a modest increment of return. Perhaps even more important, however, is the discipline that such a system provides. Having a theoretically defensible and empirically proven methodology to follow can take investor emotion out of the decision-making process, which may be the most important benefit of all.
1 Forecasting the expected return for bonds is very
straightforward. A bond is a loan with specified repayment terms. Held
to maturity, unless the seller defaults, the return is the
yield-to-maturity (YTM), which takes into account the current price, the
interest coupons, and the principal repayment at maturity. Thus, the expected return for any bond is its current yield-to-maturity.
However, for some bonds and bond indexes, if the bond gives the issuer
the right to call the bonds—which is common for corporate debt—a better
measure is the “yield-to-worst” or YTW. Schwab has a nice short
article explaining different types of bond yields here.
2 I always use exponentially-weighted moving averages when doing time-series analysis. The idea is to give more weight to more recent observations, and less weight to older observations. I think this tends to more accurately reflect how past events influence current expectations, and also it makes the analysis much less sensitive to the first observation dropping off each month as the data gets updated. The graph at right shows the weights I use for a trailing 120-month (10 year) time period.