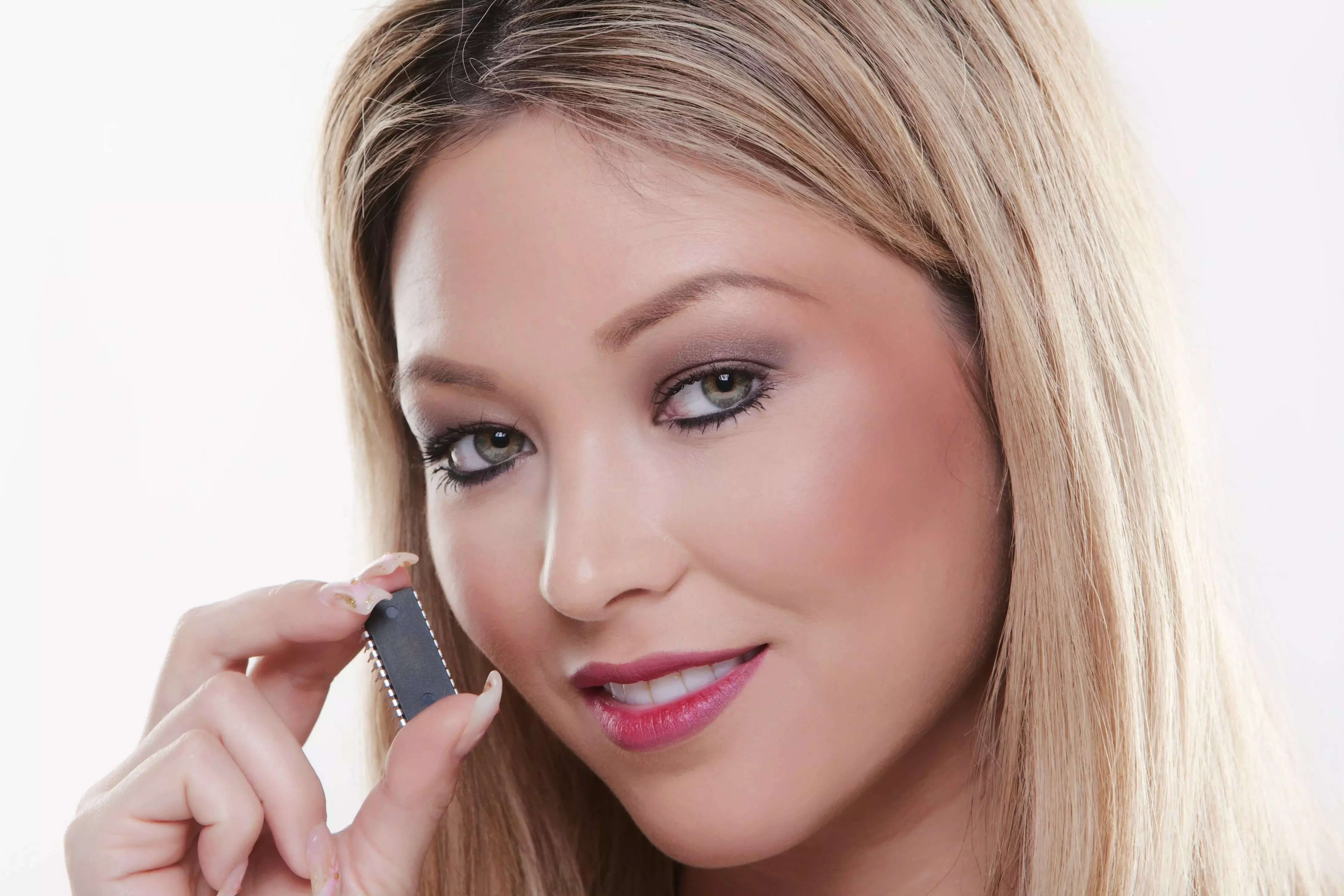
Investing in the Semiconductor Industry ETF SMH
Posted by Kevin Means, CFA on May 16, 2019 2:11:26 PM
Several characteristics have provided consistent risk-adjusted excess return for sector and industry ETFs, including value-, momentum-, quality-, and sentiment-related factors. This article highlights an industry ETF that is currently very attractive using an ETF selection model based upon such factors: VanEck Vectors Semiconductor ETF (SMH).
Sector and Industry Selection Factors
A “factor” is a mathematical way of measuring how much of a certain characteristic a security has. For example, a stock’s size is typically measured using market capitalization. A stock’s value might be measured with its price/earnings ratio. Its quality might be measured with its debt/equity ratio.
A previous article pointed out that some of the same factors (characteristics or attributes) that published academic research has found to be helpful in selecting individual stocks can be helpful in selecting sectors and industries. These factors can be grouped into the following categories:
- Value
- Momentum
- Quality
- Sentiment
In this article we will focus on one factor in each category. We will illustrate that factor’s use in sector and industry selection by showing how SMH’s “factor exposures” (or “factor loadings”) contribute to its expected risk-adjusted return in Sapient Investment’s sector and industry ETF return forecasting model. (At Sapient, we have a proprietary four-factor risk model to separate out risk-related return from risk-adjusted return.)
We base our selection of factors on published research, confirmed with our own primary research. We are able to analyze ETFs much like we would analyze stocks because our database (FactSet) aggregates stock-level information on ETF stock holdings up to the ETF level. For example, the P/E of an ETF is based upon the P/Es of the ETF’s stock holdings aggregated up according to their portfolio weights.
Standardized Factor Exposures
It may be readily apparent that stocks and ETFs with attractive value, momentum, quality, and sentiment characteristics might make good investments. The difficulty is translating that vague and intuitive sense into something more concrete and actionable. What specific factors should be used to measure each of these attractive attributes? How much emphasis should be placed on each? Should the relative weights be fixed, or should they be flexible and vary over time? Quantitative methods are very handy for answering these questions.
In general terms, the task of the quantitative analyst is to construct an investment system that will maximize risk-adjusted return in the future. It’s not that hard to construct a system that “works” based on historical data. The problem is that too often, systems too heavily trained on historical data will break down going forward in live use.
One important step that helps to prevent that is only testing factors that have a strong basis in economic theory. Markets have a strong tendency towards efficiency. Prices in efficient markets fully reflect all available information. Exceptions to that tendency must have a good explanation as to why they might exist and persist. Academics who study markets have identified several “anomalies”—exceptions to the general assumption of market efficiency. The books, journal articles, and white papers published on these market anomalies provide ideas for further primary research by the quantitative analyst. The weight of accumulated academic evidence suggests that the strongest factor anomalies are generally related to the four categories highlighted here: value, momentum, quality, and sentiment.
The statistical test most often used to measure the “payoff” to a factor (the return associated with it) is regression analysis. It may be helpful to visualize the test within an Excel spreadsheet. In Column A is the “dependent variable” that you are seeking to explain: monthly risk-adjusted returns. Each row is for a security: in this case, a sector or industry ETF. Column B contains the independent variable you believe is associated with excess return: the factor. However, rather than the raw factor, it is often transformed into a standardized factor exposure as described next.
The mechanics of regression analysis require that both the dependent and the independent variables be “normally distributed.” That is, that they fall along a nice bell-shaped curve like the one shown below:
How much an ETF has of a particular factor can be measured with the “standardized factor exposure” using the following formula:
Standardized factor exposure = (ETF factor exposure – Universe average factor exposure) Universe standard deviation of factor exposures
The graph above illustrates the standard normal distribution. The line labeled “1σ” is for 1 “sigma” the Greek letter usually used for one standard deviation. Note that in terms of percentile, a one standard deviation factor exposure is higher than 84.1% of the observations—only 15.9% are above it. The Excel regression described above calculates how much added risk-adjusted return an ETF with a one standard deviation factor exposure achieved within the month being tested.
Of course, what we really want to know is not what factor returns were in the past but what they will be in the future. Fortunately, some factors within the four categories highlighted have both a positive average payoff and also to some extent a trend-following tendency in the ebb and flow of their payoffs. Consequently, some sort of moving average of recent payoffs can be a reasonably good forecast of future expected payoffs. At Sapient, we use an exponentially weighted moving average of past returns to forecast the expected factor return over the next month.
In the sections that follow, we will drill down into one sample factor within each of the four categories, showing its historical payoff pattern within our universe of sector and industry ETFs. Currently, there are 69 sector and industry ETFs within our universe, so the sample is fairly broad. In addition, we will note the factor loading of VanEck Vectors Semiconductor ETF (SMH) with respect to each factor as of the most recent month-end (April 30, 2019), and cite the expected risk-adjusted return contribution for SMH derived from each factor. The final section will provide our overall expected return for SMH and cite a few fundamental factors affecting its investment attractiveness.
Value Factor: EBIT/EV
EBIT is earnings before interest and taxes (EBIT). It is divided by enterprise value (EV), which includes the market value of equity and the book value of debt. This value ratio is one that a private equity investor looking to purchase a whole company might use.
The graph above is a “factor graph.” It shows the cumulative return from a portfolio of sector and industry ETFs that is neutral in all respects but has a one standard deviation above average exposure to the factor. EBIT/EV has had a cumulative return of about 34% since 2008, which equates to about 3.0% per year. 3.0% does not appear very impressive on the surface. However, note that it is not cumulative total return that is being measured but the log of cumulative residual return. The distinction is vital. The vast majority of return for sector and industry ETFs is systematic return, most especially return derived from an ETF’s market beta or sensitivity to the market. Residual return is the return that is left unexplained by an ETF’s systematic risk factor sensitivities. Over the long-term, the average residual return for all of the ETFs in the sector and industry universe is zero! That’s right. There is no alpha. Overall, on average, over the long term, ETFs earn return only from their systematic risk sensitivities, especially their sensitivity to the market (or market beta).
As of April 30, 2019, VanEck Vectors Semiconductor ETF (SMH) had a standardized factor exposure to EBIT/EV that of .67, meaning that it was .67 standard deviations above the average. Based upon that factor’s exponentially weighted moving average payoff, we expected that a one standard deviation factor loading would add .18% (or 2.16% per year) to risk-adjusted return over the following month. We simply multiply SMH’s factor exposure (.67) by the expected payoff within the sector and industry ETF universe (.18%) to get the expected risk-adjusted return contribution of .12% (or 1.45% per year).
Momentum Factor: 12-Month Exp Wtd Residual Return
Most published work on momentum uses returns over the prior twelve months as the basis for the calculation. When defining momentum for individual stocks, the last month is omitted because of the strong tendency for stocks to experience short-term reversal. However, when dealing with sectors and industries, the last month is typically included because there is no evidence of short-term reversal.
Returns for various trailing time periods may be used to capture momentum effects—3,6,9, and 12 months have all been used, although 12-month trailing return is by far the most common. At Sapient Investments, our preferred methodology is to use the exponentially-weighted 12-month moving average (shown below), rather than an equal-weighted 12-month moving average.
The other important twist that we apply to measuring ETF return momentum is to use residual returns rather than total returns as the basis for the factor. Since we are attempting to forecast residual returns, it makes sense to use trailing residual return to build our factor.
The factor graph for 12-month exp. wtd. residual return (above) shows an annualized average “pure factor” residual return of 3.7% per year. In this case, what is being depicted is the cumulative log of residual return to a portfolio that is neutral in all respects but has a 1% positive exposure (rather than a one standard deviation positive exposure) to the factor (that is, a 12-month exp. wtd. residual return of 1%).
As of April 30, 2019, VanEck Vectors Semiconductor ETF (SMH) had a 12-month exponentially weighted residual return of .86%. We do not use a standardized exposure for this factor because we want to retain the raw data, including the sign. Our model indicated that we expected a payoff of 22.35% of whatever the raw factor loading was. In the case of SMH, we expect a risk-adjusted return of .19% (.86% x 22.35%) over the next month, which equates to an annualized return of 2.31%.
Quality Factor: ROIC
Return on invested capital (ROIC) is a measure of how profitable a company is. ROIC is net income divided by invested capital, which consists of the book value of both debt and equity. It differs from ROE (return on equity) in that both debt and equity are included in the denominator, so a company that uses debt instead of equity as a source of capital is not rewarded.
ROIC is indeed a powerful factor, returning an average of 5.3% per year since 2008 as shown in the factor graph above. Technology stocks tend to have the highest ROIC at present. SMH certainly fits this profile. As of April 30, 2019, VanEck Vectors Semiconductor ETF (SMH) had a standardized factor exposure to ROIC that of 2.37 meaning that it was 2.37 standard deviations above the average. Based upon that factor’s exponentially weighted moving average payoff, we expected that a one standard deviation factor loading would add .20% (or 2.40% per year) to risk-adjusted return over the following month. Since SMH had a factor exposure of 2.37, it’s added expected risk-adjusted monthly return from its exposure to ROIC was .47% (or 5.69% per year).
Sentiment Factor: EPS Estimate Revision Diffusion
The changes that analysts make in their forecast of future EPS has been shown to be a powerful predictor of future relative performance for stocks for many years. “Diffusion” is calculated as (#up - #down) / (#up + #down), which is a way of calculating %up-%down. “# up” means the number of upward analyst revisions of EPS in the last month. At Sapient Investments, we use a fairly complex way of incorporating changes in the EPS forecasts that analysts make for the underlying constituents of an ETF, combining data for the next two fiscal years.
Because it is based on changes in the estimate of future earnings, EPS estimate revision diffusion tends to be the most forward-looking factor, providing an early warning if the outlook for a sector or industry is changing. It has had a fairly consistent annualized average residual return of 3.8%, with few significant drawdowns.
As of April 30, 2019, VanEck Vectors Semiconductor ETF (SMH) had a standardized factor exposure to EPS Estimate Revision Diffusion that of .76, meaning that it was .76 standard deviations above the average. Based upon that factor’s exponentially weighted moving average payoff, we expected that a one standard deviation factor loading would add .19% (or 2.28% per year) to risk-adjusted return over the following month. SMH had a factor exposure of .76, so it’s added expected risk-adjusted monthly return from its exposure to EPS Estimate Revision Diffusion was .14% (or 1.73% per year).
Putting It All Together
The four factors analyzed above represent a subset of the factors that we currently use in our sector and industry ETF return model at Sapient Investments, but they are some of the most important ones. The overall sum of our factor-based risk-adjusted monthly return forecasts for SMH on April 30, 2019 was 1.79%. This was the highest risk-adjusted monthly return forecast within our sector and industry universe.
In addition to the factors we use to forecast risk-adjusted return, we also forecast risk-related return. For equity ETFs, this forecast is primarily driven by our return expectation for the overall stock market and the ETF’s stock market sensitivity or “beta.” Our proprietary four-factor risk model indicated that SMH had a fairly high stock market beta of 1.39 on April 30, 2019. At that time, we were forecasting a stock market return of 8.04% per year, or .67% per month, so the one-month risk-based return forecast for SMH was .93% (1.39 x .67%). Adding the risk-adjusted and risk-related forecasts together resulted in a total monthly return forecast of 2.72%.
SMH was had the highest expected return and a close substitute, iShares PHLX Semiconductor ETF (SOXX) was a close second. Clearly, semiconductor stocks were exhibiting an attractive combination of value, momentum, quality and sentiment on April 30, 2019.
Semiconductors had very high profitability and strong earnings and cash flow growth because of the enormous demand for semiconductors, which are vital for an increasing number of applications (computers, phones, cars, consumer and business electronics) with some major information technology themes providing long-term tailwinds, including the internet of things, the cloud, and artificial intelligence.
Historically, the semiconductor industry has been highly cyclical. Semiconductor spending is very economically sensitive. Because chips are light and easily shipped, production can take place in many locations. Trade frictions can disrupt supply chains and also dampen demand. This has recently been a significant risk factor for the industry.
Despite its risks, SMH appears attractively valued relative to its fundamentals, which are quite strong.
Both SMH and SOXX are relatively inexpensive, highly liquid ETFs. The expense ratios for SMH and SOXX are .35% and .47% respectively, and their respective average bid-ask spreads are .01% and .03%. SMH is slightly smaller than SOXX in terms of assets under management but is our favorite between the two because of its lower expenses of purchase and ownership.