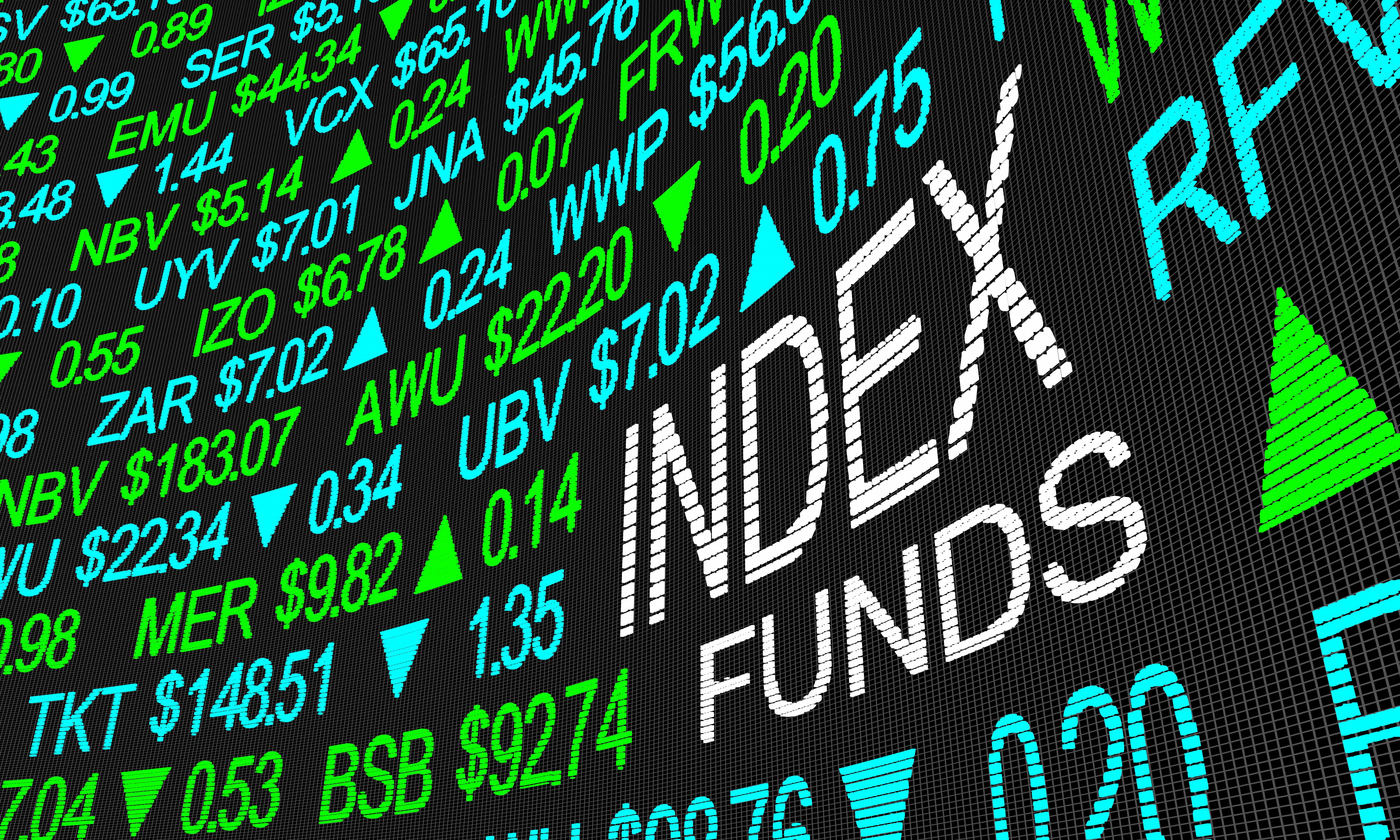
Why Invest in Index Funds?
Introduction
At Sapient Investments, we invest only in low-cost, passively-managed ETFs (with the occasional closed-end fund thrown in when the discounts to NAV become interesting). No actively managed funds. No individual stock picking.
There are two primary reasons for this. First, my expertise is in asset allocation and factor selection. Even when I was investing in individual stocks for large institutions, I was doing so merely to gain exposure to various asset classes and factors. I went out of my way to diversify away stock-specific risk as much as I could. Instead of stock selection, I wanted to focus on the “big picture” decisions—how much to allocate to stocks, and what characteristics (growth vs value, large vs small, U.S. vs international, etc.) to emphasize—that really drive long-term portfolio returns. I have written previous articles about the potential benefits of tactical asset allocation and factor selection.
Second, I am convinced that trying to add value through active stock (and bond) selection is next to impossible, not just for me but for anybody. Modern capital markets are just too efficient and competitive. The evidence demonstrating the futility of active management is overwhelming and seems to get stronger every year.
The purpose of this article is to review and summarize some of the most important research findings regarding the active vs passive debate.
A Zero-Sum Game
Many people believe that it should be fairly easy for good investment managers to “beat the market.” It seems intuitive that a fund manager who has achieved excellent returns over the long haul should be able to continue do that. The assumption is that money management is like any other field—the right combination of academic credentials, professional training, and practical experience should result in consistently positive results.
However, the reality is that it is quite rare for mutual funds to beat relevant index benchmarks, especially after subtracting transaction costs and fund expenses. As Economics Nobel Laureate Bill Sharpe pointed out in “The Arithmetic of Active Management” (1991), before costs, active management is a “zero-sum game” in which any excess return for one active manager must come at the expense of another active manager, with the sum always equal to the return of the market. Therefore, it is mathematically impossible for the average active fund to beat the market.
The following is the thought experiment in Sharpe’s article. Assume that “the market” is the sum of all passively managed assets and all actively managed assets. Since passive funds merely own a representative slice of the market, then active funds must own the remaining slice of the market. Since passive management does not incur nearly the costs that active management incurs, passive management will beat active management every time. Q.E.D. Active management is a negative sum game after costs.
Of course, this thought experiment is a simplification of reality. Assets enter the market (through IPOs and secondary offerings) and leave the market (through acquisition and bankruptcy) all the time. This activity forces even passive managers to trade. If active managers are able to anticipate this activity and trade ahead of passive managers, they may be able to add return. Also, it may be possible for certain “informed” active managers to benefit by trading against “non-informed” active managers. For example, informed managers may provide liquidity to non-informed managers, and will be compensated for this service.
And it is impossible to actually define what “the market” is, since it theoretically includes all investable assets. Some assets are more “investable” than others: micro-cap stocks, restricted international markets, direct real estate, and private equity all present issues. Therefore, no index fund can possibly invest in “the market.” These exceptions were enumerated in a 2018 FAJ article cleverly entitled “Sharpening the Arithmetic of Active Management.” But even if there are small exceptions to Sharpe’s zero-sum game, the theoretical room for active management to outperform relevant index benchmarks on this basis is extremely small—a handful of basis points at most. The typical costs of active management easily swamp these slight effects.
If mathematically the average fund must underperform the market, however, that does not necessarily mean that there aren’t superior funds out there. There are always some funds that beat their benchmarks. Indeed, some funds have outperformed their index benchmarks by an extremely wide margin over a long period of time. The problem is that such observations are likely to occur due to random chance. It is very difficult to distinguish luck from skill. Even if there are a few managers who are truly skillful enough to overcome their costs (and there may not be), the odds of selecting the right few are extremely low. Those who have studied the matter almost universally find such a search futile, as we will see below.
B.C. and A.D.
In the search for funds that are likely to outperform in the future, nearly everyone puts a lot of emphasis on past performance. Despite ubiquitous disclosures to the contrary, the implicit assumption is that past performance may be helpful in predicting future performance. But is it?
A landmark 1997 paper entitled “On Persistence in Mutual Fund Performance“ by Mark Carhart is widely thought to have settled the matter:
“Common factors in stock returns and investment expenses almost completely explain persistence in equity mutual funds' mean and risk-adjusted returns…The only significant persistence not explained is concentrated in strong underperformance by the worst-return mutual funds. The results do not support the existence of skilled or informed mutual fund portfolio managers.”
So yes, there is persistence in mutual fund performance: the worst performance is consistently among the most expensive funds where high expense ratios persist. Unfortunately, there is no persistence on the positive side of the ledger. Apart from expenses, “common factors” determine returns. Carhart used four factors: 1) market, 2) size (small-cap vs large-cap), style (value vs growth), and momentum (trailing 12-month return).
The history of the past two decades in the investment management industry can be divided into “B.C.”- before Carhart, and “A.D.” – after the resulting death of active management as a growth industry. As a recent retrospective on research published since the Carhart paper pointed out, “between 1997 and 2017, domestic equity index funds had a net cash inflow of $639 billion whereas active funds had a net outflow of $975 billion.” The explosion in the popularity of exchange-traded funds (ETFs), nearly all of which are index funds, is one indication of how the investing public’s attitudes have changed. According to ADV Ratings, the industry’s index fund powerhouse, Vanguard, is now the second largest asset manager on the planet, and Blackrock, the largest, is there primarily based on the size of its ETFs and index funds.
Separating Alpha from Beta
Risk matters. A lot. So does how you define and measure risk. Popular publications of the “horse race” variety that purport to compare active and passive funds (Morningstar’s Active/Passive Barometer, S&P Dow Jones’ SPIVA U.S. Scorecard, and Vanguard research white papers) are not terribly meaningful because they do not do a careful job of controlling for risk.
Accurately controlling for risk involves more than subtracting the returns of a benchmark index from mutual fund returns. It involves more than simply estimating a fund’s market beta and subtracting the beta-adjusted market return, as with the Capital Asset Pricing Model (CAPM) of the 1960s. The best academic research over the past several decades has shown that stock returns are driven by more than just market returns. Many possible factors have been tested, but a strong consensus has emerged around the Fama and French three factor model (1992): 1) market, 2) size (small cap vs large cap), and style (value vs growth). With the publication of Carhart’s paper in 1997, a fourth factor, momentum, was added to the standard three-factor model, and the Carhart four-factor risk model became the new academic standard.
The reason that controlling for these factors is important is that accepting more of these risk factors has been shown to add to return on average over long periods of time. That is, a systematic tilt towards smaller cap stocks, value stocks, and stocks with high trailing 12-month returns has been shown to add to return historically. Doing this does not require any judgment or skill. If your objective is to measure the value-added from active management, it is important to control for these factors.
We have seen from the discussion above that the average return of actively managed funds is certain to underperform the market. However, the more interesting question is whether it is possible to identify a few fund managers whose returns have been so high that they can be statistically proven to have added alpha (risk-adjusted excess return) after carefully controlling for risks and subtracting costs (trading costs and expense ratios).
Many of the studies seeking to answer this question do so by asking whether the entire distribution of mutual funds returns is what would be expected in the absence of any skill, or whether the extreme right tail of the return distribution is large enough to indicate skill. The two deans of academic finance, Fama and French (F&F), investigated the evidence in this way in a 2010 JOF article, “Luck versus Skill in the Cross-Section of Mutual Fund Returns.” Their conclusion does not hold out a lot of hope:
"On a practical level, our results on long-term performance say that true α [alpha] in net returns to investors is negative for most if not all active funds, including funds with strongly positive α estimates for their entire histories."
Their mutual fund return sample included all actively managed U.S. mutual funds from January 1984 to September 2006. More extreme returns are generally observed among smaller mutual funds. F&F test funds above $1 billion, $250 million, and $5 million using both gross returns (before expense ratios) and net (after expense ratios) using both their own three-factor risk model and Carhart’s four-factor risk model.
Their results show that even among funds as small as $5 million in AUM, no evidence of alpha occurs up to the 98th percentile in net returns using the three-factor risk model. Using the four-factor risk model, there is never any evidence of alpha. The very best funds, at the top of the performance heap, barely cover their expense ratios. When smaller funds are excluded, performance is even weaker.
F&F did not include index funds in their mutual fund sample. However, when tested, they found that index funds performed in line with the top 3% of all actively managed funds. Their conclusion:
"We expect that a portfolio of low cost index funds will perform about as well as a portfolio of the top three percentiles of past active winners, and better than the rest of the active fund universe."
The Ongoing Search for Mutual Fund Alpha
Some have criticized the F&F three-factor model and the Carhart four-factor model because the factors used in these models are not “investable.” The problem is that the definitions of size and style are based on returns from size- and style-sorted rankings of U.S. stocks, and not on investable indexes.
A 2019 FAJ article, “Are Passive Funds Really Superior Investments? An Investor Perspective,” seeks to remedy this possible deficiency. The authors show that a combination of five widely-available ETF types (market, large value, large growth, midcap value, and small growth) explain the returns of U.S. mutual funds as well as the academic factor-based models. They identify the combination of these five ETFs that best matches the risks of each of the actively managed U.S. mutual funds in their return sample over a 24-month period ending in December of each calendar year. Then, they compare the performance of the mutual funds against the ETF portfolio over the ensuing calendar year. They apply this methodology to all actively managed U.S. mutual funds listed by Morningstar for the 15-year period from January 2003 to December 2017. The starting period was chosen because the benchmark ETFs were all available by that date.
The ETF portfolios outperformed the corresponding active portfolios by 1.37%, which is statistically significant at the 1% level. The ETF portfolios outperformed in 78% of the cases. When loads are included in active fund returns, ETFs outperformed 90% of the time. The ETF portfolios also had lower average standard deviations (risk).
Some active funds did outperform their matching ETF portfolios. The relevant question then becomes, would it have been possible to predict which funds would outperform ahead of time? The authors examine five common selection criteria:
1. Past alpha
2. Past expenses
3. Past turnover
4. Morningstar rating
5. R2 and R2 plus alpha
Applying the first three variables (high alpha, low expenses, and low turnover) did not produce returns for active funds that were better than those for the passive ETFs in the top decile or any other decile. The only one of these three criteria that produced a significant rank correlation was past expenses. Morningstar five-star funds performed better than other star ratings, but their ETFs still outperformed them. R2 and R2 plus alpha are statistically robust ways of measuring “active share,” or the degree to which actively managed portfolios have weights that differ from their benchmark indexes. However, even the highest-risk group (lowest R2 and R2 plus alpha and therefore the highest active share) underperformed their ETF-matched portfolios.
Thus, not only did risk-matching ETF portfolios vastly outperform the average returns of actively-managed mutual funds, but no method was found for identifying which funds might be the few that outperformed their ETF portfolios in the future, indicating that these were random events.
Summary and Conclusions
- Active management is a zero-sum game before costs and a negative sum game after costs.
- The only consistent predictor of future mutual fund return rankings is the expense ratio—expensive funds are always the worst performers over the long-term.
- Not only does the average active fund underperform its benchmark index, but nearly all funds do on average over time.
- Studies that carefully control for risk show that the few occurrences of very high performing funds arise no more often than would be explained by chance.
- A portfolio of low-cost index funds is expected to perform about as well as the top 3% of risk-matched actively managed funds.
- There is no selection methodology that can be used to identify future outperformers.